A Review of Progress and Challenges in Knowledge-Based, Machine Learning, and Crowd-Sourced Approaches to Commonsense Reasoning in AI
Abstract
Commonsense reasoning, the cornerstone of human-like artificial intelligence (AI), refers to the ability of systems to make contextually relevant inferences about mundane situations, simulating human intuitive intelligence. In spite of major progress, AI research is still divided into two major paradigms: knowledge-based systems that symbolically represent commonsense knowledge by explicit encoding of structured knowledge and machine learning (ML)-based approaches that implicitly learn commonsense by extracting patterns from vast textual corpora. Here, we discuss both paradigms in depth side by side, contrasting their relative merits and intrinsic weaknesses. We also cover hybrid crowd-sourcing approaches, which combine human-generated observations to produce comprehensive, open knowledge bases, and thus augment the limitations of purely automatic techniques. The paper also identifies qualitative reasoning as a paradigmatic example of mathematically precise but intuitive physical and spatial commonsense modeling, and its usefulness in the presence of incomplete or incorrect data. Some of the key challenges still lie in the seamless integration of formal logic-based reasoning, cognitive-inspired models, and data-driven learning approaches. These involve knowledge breadth management, exception and context-dependent information management, explainability guarantees, and reducing biases in crowd-sourced or learned datasets. The paper concludes by presenting strategic suggestions on how to build strong and scalable commonsense reasoning systems that necessitate integration of symbolic reasoning, statistical learning, qualitative models, and human-generated knowledge with the aim of finally making AI systems to be capable of understanding and interacting with real-world environments' complexities.
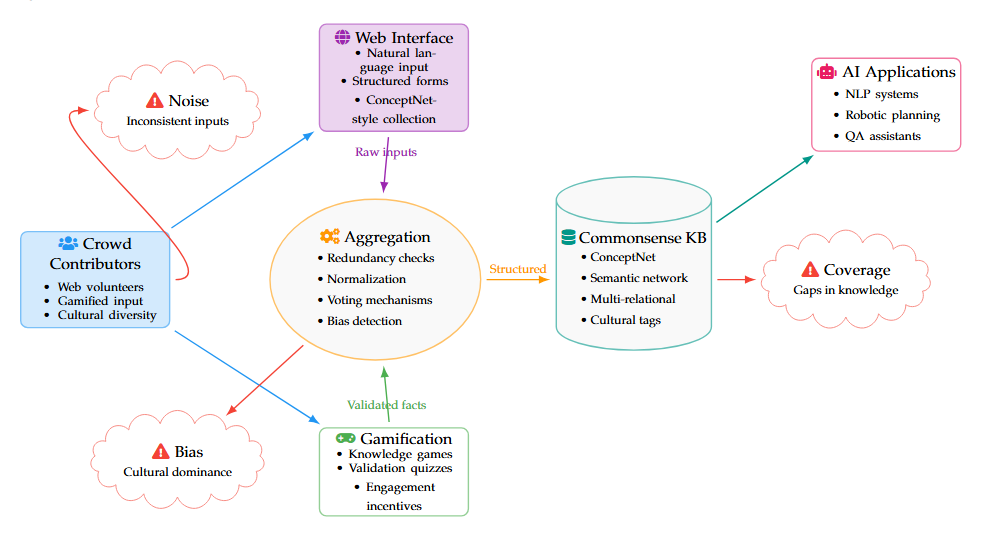